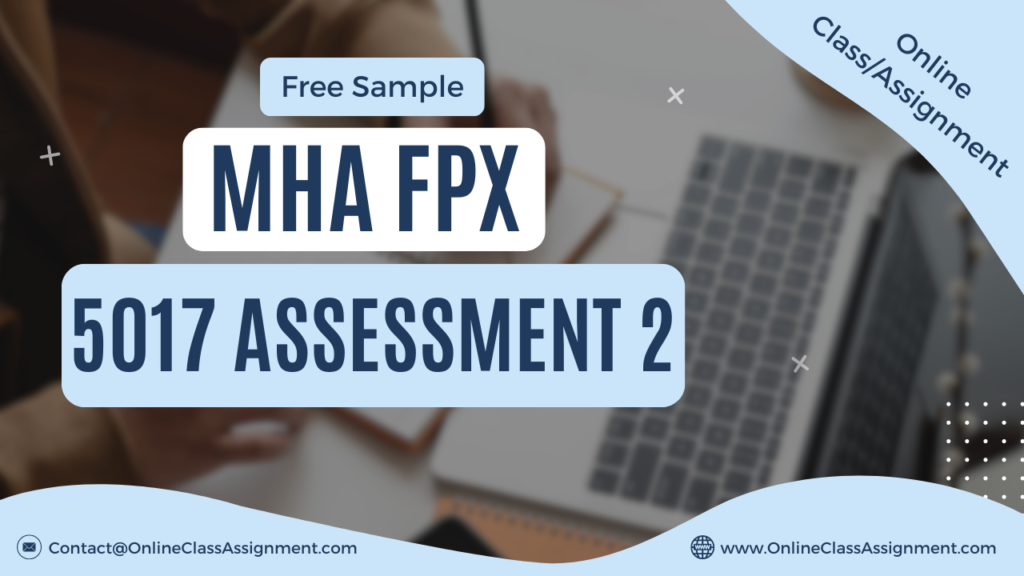
MHA FPX 5017 Assessment 2 Hypothesis Testing for Differences Between Groups
Student Name
Capella University
MHA-FPX 5017 Data Analysis for Health Care Decisions
Prof. Name
Date
Hypothesis Testing for Group Differences
In inferential statistics, populations of individuals are subject to analysis and testing using hypothesis testing, which aids in comparing datasets and facilitating conclusive decision-making. This process revolves around two types of hypotheses: null and alternative. The null hypothesis posits no significant difference in data compared side by side, while the alternative hypothesis suggests substantial differences within the dataset (Hacker & Hatemi-J, 2022).
Research Scenario
The comparison of productivity levels between two clinics, Clinic 1 and Clinic 2, is conducted using null and alternative hypotheses. The null hypothesis (H0) proposes no difference in productivity between the two clinics, while the alternative hypothesis (Ha) supports differences in productivity. These hypotheses can be expressed as equations: [H_0: \text{Clinic 1} = \text{Clinic 2}] [H_a: \text{Clinic 1} \neq \text{Clinic 2}].
Selection of Statistical Tests
The determination of a normal distribution between the samples guides the choice of tests. A symmetric distribution ensures symmetrical data presentation, while the current asymmetric appearance signifies unequal variances, favoring the Wilcoxon Signed-Rank test (Chang & Perron, 2017).
Two-Sample t-tests Results
Both samples possess a sufficient sample size (n = 100) warranting an independent t-test for estimating the normal distribution. Presented below are two independent t-tests, one assuming equal variances and the other assuming unequal variances.
Table 1: Two-Sample t-test Assuming Equal Variances
Clinic 1 | Clinic 2 | |
---|---|---|
Mean | 124.32 | 145.03 |
Variance | 2188.543 | 1582.514 |
Observations | 100 | 100 |
Pooled Variance | 1885.529 | – |
Hypothesized Mean Difference | 0 | – |
df | 198 | – |
t Stat | -3.37247 | – |
P(T<=t) one-tail | 0.000448 | – |
t Critical one-tail | 1.65258 | – |
P(T<=t) two-tail | 0.000896 | – |
t Critical two-tail | 1.972017 | – |
Table 2: Two-Sample t-test Assuming Unequal Variances
Clinic 1 | Clinic 2 | |
---|---|---|
Mean | 124.32 | 145.03 |
Variance | 2188.543 | 1582.514 |
Observations | 100 | 100 |
Pooled Variance | 1885.529 | – |
Hypothesized Mean Difference | 0 | – |
df | 193 | – |
t Stat | -3.37247 | – |
P(T<=t) one-tail | 0.00045 | – |
t Critical one-tail | 1.652787 | – |
P(T<=t) two-tail | 0.0009 | – |
t Critical two-tail | 1.972332 | – |
Interpretation and Recommendation
Clinic 2 exhibits a higher mean than Clinic 1 in both scenarios, indicating better performance. With p-values less than the significance level (α = 0.05), the null hypothesis is rejected. Consequently, Clinic 1’s patient visit ratios differ from Clinic 2’s based on the data.
MHA FPX 5017 Assessment 2 Hypothesis Testing for Differences Between Groups
Recommendation
According to the data, Clinic 2 appears to outperform Clinic 1, albeit with a relatively close performance. Remedial actions for underperforming clinics involve analyzing clinical workflows, scheduling and booking software, staff education, billing, and coding practices. A comprehensive analysis identifies deficient areas, enabling administrators to formulate data-driven recommendations for enhancing clinic performance (Aspalter, 2023).
References
Aspalter, C. (2023). Evaluating and Measuring Exactly the Distances between Aggregate Health Performances: A Global Health Data and Welfare Regime Analysis. Social Development Issues, 45(1), 1-36. Link
Chang, S. Y., & Perron, P. (2017). Fractional Unit Root Tests Allowing for a Structural Change in Trend under Both the Null and Alternative Hypotheses. Econometrics, 5(1), 5. DOI
MHA FPX 5017 Assessment 2 Hypothesis Testing for Differences Between Groups
Hacker, R. S., & Hatemi-J, A. (2022). Model selection in time series analysis: using information criteria as an alternative to hypothesis testing. Journal of Economic Studies, 49(6), 1055-1075. DOI
Get Capella University Free MHA Samples
MHA FPX 5010
Deprecated: Creation of dynamic property CatListDisplayer::$parent is deprecated in /home3/fikmtkmy/public_html/wp-content/plugins/list-category-posts/include/lcp-catlistdisplayer.php on line 24
Deprecated: Creation of dynamic property CatList::$saved_wp_query is deprecated in /home3/fikmtkmy/public_html/wp-content/plugins/list-category-posts/include/lcp-catlist.php on line 55
Deprecated: Creation of dynamic property CatList::$saved_post is deprecated in /home3/fikmtkmy/public_html/wp-content/plugins/list-category-posts/include/lcp-catlist.php on line 57
Deprecated: preg_replace(): Passing null to parameter #3 ($subject) of type array|string is deprecated in /home3/fikmtkmy/public_html/wp-includes/formatting.php on line 4784
Deprecated: preg_replace(): Passing null to parameter #3 ($subject) of type array|string is deprecated in /home3/fikmtkmy/public_html/wp-includes/formatting.php on line 4784
Deprecated: preg_replace(): Passing null to parameter #3 ($subject) of type array|string is deprecated in /home3/fikmtkmy/public_html/wp-includes/formatting.php on line 4784
MHA FPX 5020
Deprecated: Creation of dynamic property CatListDisplayer::$parent is deprecated in /home3/fikmtkmy/public_html/wp-content/plugins/list-category-posts/include/lcp-catlistdisplayer.php on line 24
Deprecated: Creation of dynamic property CatList::$saved_wp_query is deprecated in /home3/fikmtkmy/public_html/wp-content/plugins/list-category-posts/include/lcp-catlist.php on line 55
Deprecated: Creation of dynamic property CatList::$saved_post is deprecated in /home3/fikmtkmy/public_html/wp-content/plugins/list-category-posts/include/lcp-catlist.php on line 57
Deprecated: preg_replace(): Passing null to parameter #3 ($subject) of type array|string is deprecated in /home3/fikmtkmy/public_html/wp-includes/formatting.php on line 4784
Deprecated: preg_replace(): Passing null to parameter #3 ($subject) of type array|string is deprecated in /home3/fikmtkmy/public_html/wp-includes/formatting.php on line 4784
Deprecated: preg_replace(): Passing null to parameter #3 ($subject) of type array|string is deprecated in /home3/fikmtkmy/public_html/wp-includes/formatting.php on line 4784
Deprecated: preg_replace(): Passing null to parameter #3 ($subject) of type array|string is deprecated in /home3/fikmtkmy/public_html/wp-includes/formatting.php on line 4784
Deprecated: preg_replace(): Passing null to parameter #3 ($subject) of type array|string is deprecated in /home3/fikmtkmy/public_html/wp-includes/formatting.php on line 4784
MHA FPX 5042
Deprecated: Creation of dynamic property CatListDisplayer::$parent is deprecated in /home3/fikmtkmy/public_html/wp-content/plugins/list-category-posts/include/lcp-catlistdisplayer.php on line 24
Deprecated: Creation of dynamic property CatList::$saved_wp_query is deprecated in /home3/fikmtkmy/public_html/wp-content/plugins/list-category-posts/include/lcp-catlist.php on line 55
Deprecated: Creation of dynamic property CatList::$saved_post is deprecated in /home3/fikmtkmy/public_html/wp-content/plugins/list-category-posts/include/lcp-catlist.php on line 57
Deprecated: preg_replace(): Passing null to parameter #3 ($subject) of type array|string is deprecated in /home3/fikmtkmy/public_html/wp-includes/formatting.php on line 4784
Deprecated: preg_replace(): Passing null to parameter #3 ($subject) of type array|string is deprecated in /home3/fikmtkmy/public_html/wp-includes/formatting.php on line 4784
Deprecated: preg_replace(): Passing null to parameter #3 ($subject) of type array|string is deprecated in /home3/fikmtkmy/public_html/wp-includes/formatting.php on line 4784
Deprecated: preg_replace(): Passing null to parameter #3 ($subject) of type array|string is deprecated in /home3/fikmtkmy/public_html/wp-includes/formatting.php on line 4784
MHA FPX 5040
Deprecated: Creation of dynamic property CatListDisplayer::$parent is deprecated in /home3/fikmtkmy/public_html/wp-content/plugins/list-category-posts/include/lcp-catlistdisplayer.php on line 24
Deprecated: Creation of dynamic property CatList::$saved_wp_query is deprecated in /home3/fikmtkmy/public_html/wp-content/plugins/list-category-posts/include/lcp-catlist.php on line 55
Deprecated: Creation of dynamic property CatList::$saved_post is deprecated in /home3/fikmtkmy/public_html/wp-content/plugins/list-category-posts/include/lcp-catlist.php on line 57
Deprecated: preg_replace(): Passing null to parameter #3 ($subject) of type array|string is deprecated in /home3/fikmtkmy/public_html/wp-includes/formatting.php on line 4784
Deprecated: preg_replace(): Passing null to parameter #3 ($subject) of type array|string is deprecated in /home3/fikmtkmy/public_html/wp-includes/formatting.php on line 4784
Deprecated: preg_replace(): Passing null to parameter #3 ($subject) of type array|string is deprecated in /home3/fikmtkmy/public_html/wp-includes/formatting.php on line 4784
Deprecated: preg_replace(): Passing null to parameter #3 ($subject) of type array|string is deprecated in /home3/fikmtkmy/public_html/wp-includes/formatting.php on line 4784
MHA FPX 5016
Deprecated: Creation of dynamic property CatListDisplayer::$parent is deprecated in /home3/fikmtkmy/public_html/wp-content/plugins/list-category-posts/include/lcp-catlistdisplayer.php on line 24
Deprecated: Creation of dynamic property CatList::$saved_wp_query is deprecated in /home3/fikmtkmy/public_html/wp-content/plugins/list-category-posts/include/lcp-catlist.php on line 55
Deprecated: Creation of dynamic property CatList::$saved_post is deprecated in /home3/fikmtkmy/public_html/wp-content/plugins/list-category-posts/include/lcp-catlist.php on line 57
Deprecated: preg_replace(): Passing null to parameter #3 ($subject) of type array|string is deprecated in /home3/fikmtkmy/public_html/wp-includes/formatting.php on line 4784
Deprecated: preg_replace(): Passing null to parameter #3 ($subject) of type array|string is deprecated in /home3/fikmtkmy/public_html/wp-includes/formatting.php on line 4784
Deprecated: preg_replace(): Passing null to parameter #3 ($subject) of type array|string is deprecated in /home3/fikmtkmy/public_html/wp-includes/formatting.php on line 4784
Deprecated: preg_replace(): Passing null to parameter #3 ($subject) of type array|string is deprecated in /home3/fikmtkmy/public_html/wp-includes/formatting.php on line 4784
MHA FPX 5012
Deprecated: Creation of dynamic property CatListDisplayer::$parent is deprecated in /home3/fikmtkmy/public_html/wp-content/plugins/list-category-posts/include/lcp-catlistdisplayer.php on line 24
Deprecated: Creation of dynamic property CatList::$saved_wp_query is deprecated in /home3/fikmtkmy/public_html/wp-content/plugins/list-category-posts/include/lcp-catlist.php on line 55
Deprecated: Creation of dynamic property CatList::$saved_post is deprecated in /home3/fikmtkmy/public_html/wp-content/plugins/list-category-posts/include/lcp-catlist.php on line 57
Deprecated: preg_replace(): Passing null to parameter #3 ($subject) of type array|string is deprecated in /home3/fikmtkmy/public_html/wp-includes/formatting.php on line 4784
Deprecated: preg_replace(): Passing null to parameter #3 ($subject) of type array|string is deprecated in /home3/fikmtkmy/public_html/wp-includes/formatting.php on line 4784
Deprecated: preg_replace(): Passing null to parameter #3 ($subject) of type array|string is deprecated in /home3/fikmtkmy/public_html/wp-includes/formatting.php on line 4784
Deprecated: preg_replace(): Passing null to parameter #3 ($subject) of type array|string is deprecated in /home3/fikmtkmy/public_html/wp-includes/formatting.php on line 4784
MHA FPX 5014
Deprecated: Creation of dynamic property CatListDisplayer::$parent is deprecated in /home3/fikmtkmy/public_html/wp-content/plugins/list-category-posts/include/lcp-catlistdisplayer.php on line 24
Deprecated: Creation of dynamic property CatList::$saved_wp_query is deprecated in /home3/fikmtkmy/public_html/wp-content/plugins/list-category-posts/include/lcp-catlist.php on line 55
Deprecated: Creation of dynamic property CatList::$saved_post is deprecated in /home3/fikmtkmy/public_html/wp-content/plugins/list-category-posts/include/lcp-catlist.php on line 57
Deprecated: preg_replace(): Passing null to parameter #3 ($subject) of type array|string is deprecated in /home3/fikmtkmy/public_html/wp-includes/formatting.php on line 4784
Deprecated: preg_replace(): Passing null to parameter #3 ($subject) of type array|string is deprecated in /home3/fikmtkmy/public_html/wp-includes/formatting.php on line 4784
Deprecated: preg_replace(): Passing null to parameter #3 ($subject) of type array|string is deprecated in /home3/fikmtkmy/public_html/wp-includes/formatting.php on line 4784
Deprecated: preg_replace(): Passing null to parameter #3 ($subject) of type array|string is deprecated in /home3/fikmtkmy/public_html/wp-includes/formatting.php on line 4784
Deprecated: preg_replace(): Passing null to parameter #3 ($subject) of type array|string is deprecated in /home3/fikmtkmy/public_html/wp-includes/formatting.php on line 4784
MHA FPX 5017
Deprecated: Creation of dynamic property CatListDisplayer::$parent is deprecated in /home3/fikmtkmy/public_html/wp-content/plugins/list-category-posts/include/lcp-catlistdisplayer.php on line 24
Deprecated: Creation of dynamic property CatList::$saved_wp_query is deprecated in /home3/fikmtkmy/public_html/wp-content/plugins/list-category-posts/include/lcp-catlist.php on line 55
Deprecated: Creation of dynamic property CatList::$saved_post is deprecated in /home3/fikmtkmy/public_html/wp-content/plugins/list-category-posts/include/lcp-catlist.php on line 57
Deprecated: preg_replace(): Passing null to parameter #3 ($subject) of type array|string is deprecated in /home3/fikmtkmy/public_html/wp-includes/formatting.php on line 4784
Deprecated: preg_replace(): Passing null to parameter #3 ($subject) of type array|string is deprecated in /home3/fikmtkmy/public_html/wp-includes/formatting.php on line 4784
Deprecated: preg_replace(): Passing null to parameter #3 ($subject) of type array|string is deprecated in /home3/fikmtkmy/public_html/wp-includes/formatting.php on line 4784
Deprecated: preg_replace(): Passing null to parameter #3 ($subject) of type array|string is deprecated in /home3/fikmtkmy/public_html/wp-includes/formatting.php on line 4784